Bayesian Belief Propagation (BBP) was formulated by Judea Pearl, who later authored Probabilistic Reasoning in Intelligent Systems. It appears to be a system for determining unknown probabilities within certain types of complex systems. Let's start with a review of a very simple probability model.
Much of early probability work was formulated in terms of a pair of ordinary gambling dice, six-sided cubes with faces numbered one through six. Thrown many times, they make a nice distribution pattern of numbers two through twelve. It can be shown with a little math and logic that there are percentages that are expected for each of these numbers. If you make a large number of dice throws, say more than 100, and you get results that differ by very much from the expected distribution of results, you may be looking at one of two things. It is possible that you have a distribution skewed purely by chance. On the other hand, you might want to investigate whether your dice and throwing systems are physically constructed to be truly random in the results they give.
Now imagine a black box, say the size of a shoe box. If you shake the box and then stop shaking, it gives you a number. In fact, imagine a room with a variety of such boxes. You shake them, keeping tracks of the resulting numbers for each. If one box consistently gives numbers between 2 and 12 inclusive, and the frequencies for each number match the pattern that we believe two dice would give, then we can conclude that the internal mechanism of the box, whatever it is, has a probability distribution that is created by the same logic that creates the dice probability distribution. There might even be a tiny pair of nano-dice inside.
In computer programming we tend to think in purely causal mechanics. If something does not happen as expected, we have a bug in our program. But in the case of living groups of biological neurons we cannot (so far) look at the inner mechanics of each neuron while watching how the whole group operates. However, if we can control the inputs and read the outputs of a system, then, if we can do the math, we might figure out the probabilities of individual neurons firing given known inputs from the other neurons in the system.
Formally, BBP allows us to calculate the probability characteristics for nodes we can't observe directly, if we can observe enough of the rest of the system. A node does not need to be a neuron. In an AI system, a node is a processing unit with inputs, outputs, or both, that connect it to the system of nodes in question. In our case the nodes are part of a Bayesian network, also known as a belief network. Communication (or messaging) within the network is in terms of probabilities. (See also Bayesian probability)
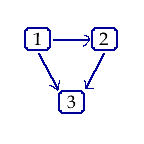
Think of a network of three people, where each of the people represents a node. Let there be a piece of paper we will call the message (it is blank; the paper is the message, in this case). Let each person have a single die and the rule: if you have the message, throw the die. If it comes up a one, a two, or a three, pass the message to the left. Otherwise, pass it to the right. If we watch the game progress, the message moves around or back and forth in a random pattern. However, it is not a Bayesian network, because it is cyclical. Bayesian networks are not cyclic. Messages more only in one direction.
Now start a series of games in which the message is always handed to person 1 (node 1). Person 1 throws the die and passes the message to person 2 if the result is 3 or less, and to person 3 if the result is 3 or more. Person 2 also throws a die and simply holds the message if the result is 3 or less, but passes if to person 3 if the result is 3 or more. When person 3 gets a message the game is over.
It is a simple game and a tad boring, but if we focus on the propagation time, the number of die throws from the beginning of a game until the end of a game, we can see a set of variations. Over the long run they should form a probability distribution of the times.
Now change the rules slightly. Say person 2 forwards the message if the die comes out 2 or higher. The range of possible results does not change, but the probability distribution will.
Now suppose you know about this type of game, but you can't see the game because it is in a tent. You see a message handed in, and can time how long it is before the message is handed back out by person 3.
By keeping track of the times, you can get the probability distribution. That should tell you the exact rules of the game being played, including the probability that person 1 will give the message directly to person 3, and how long person 2 might keep the message before passing it.
In more complex networks, you need to pin down the probabilities at more of the nodes, if you want to be able to characterize the probability rules for an unknown node.
Which is what the BBP algorithm is supposed to do. It calculates the probabilities for an unobservable node based on the observed nodes.